A Semi-Supervised Learning Approach for Tissue Semantic Segmentation in Whole Slide Images
Document Type
Article
Publication Title
IEEE Access
Abstract
Tissue semantic segmentation from Hematoxylin and Eosin (H&E)-stained Whole Slide Images (WSIs) is a highly effective technique in medical image analysis that has significantly impacted clinical practice and scientific research. Accurate delineation of tissue boundaries allows for precise identification of diseases and the formulation of successful treatment strategies. Nevertheless, the process for developing such a system presents considerable obstacles because of the intrinsic diversity in tissue structure, the existence of interference, and the extensive array of colour distributions. Several studies have investigated various deep learning architectural frameworks for the purpose of tissue segmentation. However, deep learning algorithms require a significant volume of data in order to successfully learn complex patterns and characteristics. The lack of consistent large-scale datasets hinders the progress of these models. To this end, semi-supervised learning strategies can facilitate accurate segmentation of WSIs in the absence of large annotated datasets. Moreover, there is a pressing need for the advancement of these technologies. The objective of this study is to design a semi-supervised learning technique for tissue semantic segmentation from WSI. The work employs a hybrid strategy that combines pseudo-label generation and consistency regularization to efficiently utilize the partially labeled dataset. Additionally, a novel pseudo-label refinement module is proposed to reduce the frequency of noisy pseudo-labels. Furthermore, there is a requirement for the development of a proficient feature extractor that can efficiently extract rich features from the images. This study tackles this problem by introducing a deep-learning segmentation framework, TS-Net, which can accurately segment tissue from histopathological images even with small datasets. The proposed model uses convolution and transformer layers to efficiently extract supplementary information from the image. The performance of the proposed model is comprehensively assessed both qualitatively and quantitatively using a publicly available dataset. The evaluation showcases competitive outcomes when compared to the other techniques currently available.
First Page
120482
Last Page
120497
DOI
10.1109/ACCESS.2024.3438568
Publication Date
1-1-2024
Recommended Citation
Rashmi, R.; Sudhamsh, G. V.S.; and Girisha, S., "A Semi-Supervised Learning Approach for Tissue Semantic Segmentation in Whole Slide Images" (2024). Open Access archive. 10815.
https://impressions.manipal.edu/open-access-archive/10815
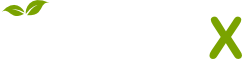
- Citations
- Citation Indexes: 2
- Usage
- Abstract Views: 1
- Captures
- Readers: 13
- Mentions
- News Mentions: 1