Knowledge-based recommendation system using semantic web rules based on Learning styles for MOOCs
Document Type
Article
Publication Title
Cogent Engineering
Abstract
With web-based education and Technology Enhanced Learning (TEL) assuming new importance, there has been a shift towards Massive Open Online Courses (MOOC) platforms owing to their openness and flexible “on-the-go” nature. The previous decade has seen tremendous research in the field of Adaptive E-Learning Systems but work in the field of personalization in MOOCs is still a promising avenue. This paper aims to discuss the scope of said personalization in a MOOC environment along with proposing an approach to build a knowledge-based recommendation system that uses multiple domain ontologies and operates on semantically related usage data. The recommendation system employs cluster-based collaborative filtering in conjunction with rules written in the Semantic Web Rule Language (SWRL) and thus is truly a hybrid recommendation system. It has at its core, clusters of learners which are segregated using predicted learning style in accordance with the Felder Silverman Learning Style Model (FSLSM) through the detection of tracked usage parameters. Recommendations are made to the granularity of internal course elements along with learning path recommendation and provided general learning tips and suggestions. The study is concluded with an observed positive trend in the learning experience of participants, gauged through click-through log and explicit feedback forms. In addition, the impact of recommendation is statistically analyzed and used to improve the recommendations.
DOI
10.1080/23311916.2021.2022568
Publication Date
1-1-2022
Recommended Citation
Agarwal, Abhinav; Mishra, Divyansh Shankar; and Kolekar, Sucheta V., "Knowledge-based recommendation system using semantic web rules based on Learning styles for MOOCs" (2022). Open Access archive. 5152.
https://impressions.manipal.edu/open-access-archive/5152
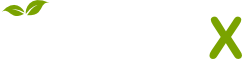
- Citations
- Citation Indexes: 45
- Usage
- Abstract Views: 2
- Captures
- Readers: 134
- Mentions
- News Mentions: 1