Automotive intelligence: Unleashing the potential of AI beyond advance driver assisting system, a comprehensive review
Document Type
Article
Publication Title
Computers and Electrical Engineering
Abstract
Big data, AI, and machine learning have ushered in a transformative era in computer processing and application. AI's capacity to emulate human cognition spans a spectrum of industries, from rudimentary tasks to intricate decision-making processes. Projections indicate that within a short span, AI technology will bestow computers with human-like capabilities, rendering it indispensable. This advancement has substantially bolstered industry autonomy, fortifying security and safety through the integration of electronics, internet connectivity, advanced algorithms, and cutting-edge processing chips. In this context, Automated Guided Vehicles (AGVs) leverage AI-powered systems and sensors to optimize performance and proactively thwart potential failures. Going beyond Advanced Driver Assistance Systems (ADAS), this review scrutinizes the broader spectrum of AI applications. Specifically, it synthesizes pertinent studies encompassing AI's impact on car emissions, predictive maintenance, security, and the realm of connected vehicles. While AGVs can utilize AI algorithms to chart emission-efficient routes and engineer energy-conservative vehicles, AI, through diverse algorithms, proficiently anticipates component failures, substantially enhancing the cost-effectiveness of predictive maintenance and bolstering vehicle reliability. Moreover, AI integrated with sensor technology safeguards vehicles against a spectrum of threats. Neural networks and machine learning further automate security measures for both cargo and passengers. Concurrently, AI and machine learning fortify traffic control and enhance vehicle communication within connected vehicle frameworks, albeit necessitating fortifications in data security, encryption, and system efficiency. This survey paper comprehensively covers the expanse of automotive AI and machine learning applications, extending beyond ADAS. It underscores that the evolving mobility landscape necessitates a thorough evaluation of network architecture, connectivity, and performance metrics, encompassing AGV technology, networking protocols, and swarm dynamics. In this vein, the paper advocates for experts to address existing literature gaps and proactively enhance automotive systems.
DOI
10.1016/j.compeleceng.2024.109237
Publication Date
7-1-2024
Recommended Citation
Rana, Kapileswar and Khatri, Narendra, "Automotive intelligence: Unleashing the potential of AI beyond advance driver assisting system, a comprehensive review" (2024). Open Access archive. 6496.
https://impressions.manipal.edu/open-access-archive/6496
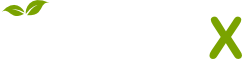
- Citations
- Citation Indexes: 15
- Usage
- Abstract Views: 12
- Captures
- Readers: 192
- Mentions
- News Mentions: 1