LeafSpotNet: A deep learning framework for detecting leaf spot disease in jasmine plants
Document Type
Article
Publication Title
Artificial Intelligence in Agriculture
Abstract
Leaf blight spot disease, caused by bacteria and fungi, poses a threat to plant health, leading to leaf discoloration and diminished agricultural yield. In response, we present a MobileNetV3 based classifier designed for the Jasmine plant, leveraging lightweight Convolutional Neural Networks (CNNs) to accurately identify disease stages. The model integrates depth wise convolution layers and max pool layers for enhanced feature extraction, focusing on crucial low level features indicative of the disease. Through preprocessing techniques, including data augmentation with Conditional GAN and Particle Swarm Optimization for feature selection, the classifier achieves robust performance. Evaluation on curated datasets demonstrates an outstanding 97% training accuracy, highlighting its efficacy. Real world testing with diverse conditions, such as extreme camera angles and varied lighting, attests to the model's resilience, yielding test accuracies between 94% and 96%. The dataset's tailored design for CNN based classification ensures result reliability. Importantly, the model's lightweight classification, marked by fast computation time and reduced size, positions it as an efficient solution for real time applications. This comprehensive approach underscores the proposed classifier's significance in addressing leaf blight spot disease challenges in commercial crops.
First Page
1
Last Page
18
DOI
10.1016/j.aiia.2024.02.002
Publication Date
6-1-2024
Recommended Citation
V, Shwetha; Bhagwat, Arnav; and Laxmi, Vijaya, "LeafSpotNet: A deep learning framework for detecting leaf spot disease in jasmine plants" (2024). Open Access archive. 6543.
https://impressions.manipal.edu/open-access-archive/6543
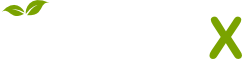
- Citations
- Citation Indexes: 19
- Usage
- Abstract Views: 7
- Captures
- Readers: 77
- Mentions
- News Mentions: 1