Optimizing Building Energy Management with Deep Reinforcement Learning for Smart and Sustainable Infrastructure
Document Type
Article
Publication Title
Journal of Machine and Computing
Abstract
This study develops a new technique for optimising Energy Consumption (EC) and occupant satisfaction in business centres using Building Energy Management Systems (BEMS) that implement Deep Reinforcement Learning (DRL). Energy Management Models (EMM) are growing increasingly advanced and vital for intelligent power systems due to the growing demand for energy efficiency and the adoption of Renewable Energy Sources (RES), which are subject to variability. Flawed energy Consumption (EC) and problems are typical effects of traditional BEMS due to their unpredictability and failure to adapt to new environments. In this intended investigation, a DRL framework is demonstrated that may evolve its decision-making in real-time to control energy savings, electricity, and HVAC through input from the environment in which it operates. A pair of significant metrics, namely the cost of energy and room temperature stability, are employed to assess the effectiveness of the model compared to that provided by conventional rule-driven and predictive control systems. As investigated with different baseline models, the experimental findings proved that the DRL approach significantly reduced the cost of electricity while maintaining stable levels of comfort.
First Page
381
Last Page
391
DOI
10.53759/7669/jmc202404036
Publication Date
4-1-2024
Recommended Citation
Alsharafa, Nabeel S.; Suguna, R.; Krishna, Raguru Jaya; and Sonthi, Vijaya Krishna, "Optimizing Building Energy Management with Deep Reinforcement Learning for Smart and Sustainable Infrastructure" (2024). Open Access archive. 6679.
https://impressions.manipal.edu/open-access-archive/6679
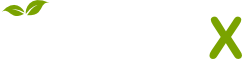
- Citations
- Citation Indexes: 1
- Usage
- Abstract Views: 38
- Captures
- Readers: 5
- Mentions
- News Mentions: 1