Neural Network-Based Hammerstein Model Identification of a Lab-Scale Batch Reactor
Document Type
Article
Publication Title
ACS Omega
Abstract
This paper focuses on two types of neural network-based Hammerstein model identification methods for the acrylamide polymerization reaction of a batch reactor process. The first neural-based identification type formulates the weights of the multilayer network directly as parameters of the nonlinear static and linear dynamic blocks of the Hammerstein model and trains the weights using a gradient-based backpropagation algorithm. In the second identification type, the nonlinear static block of the Hammerstein model is framed as a single hidden-layer feedforward network and both nonlinear and linear block parameters are trained using an extreme learning machine, where the training procedure is exempted from gradient calculation. The primary focus of the paper is neural-based model identification of a complex nonlinear system, which facilitates ease of linear/nonlinear controller design with good learning speed and less computations. A future work toward the machine learning-based nonlinear model predictive controller implementation using the Jetson Orin Nano board is also described.
First Page
1762
Last Page
1769
DOI
10.1021/acsomega.3c05406
Publication Date
1-9-2024
Recommended Citation
Balakrishnan, Murugan; Rajendran, Vinodha; Prajwal, Shettigar J.; and Indiran, Thirunavukkarasu, "Neural Network-Based Hammerstein Model Identification of a Lab-Scale Batch Reactor" (2024). Open Access archive. 6960.
https://impressions.manipal.edu/open-access-archive/6960
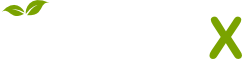
- Citations
- Citation Indexes: 6
- Usage
- Abstract Views: 2
- Captures
- Readers: 5
- Mentions
- News Mentions: 1