A Lightweight Meta-Ensemble Approach for Plant Disease Detection Suitable for IoT-Based Environments
Document Type
Article
Publication Title
IEEE Access
Abstract
By providing food to billions of people, agriculture contributes significantly to the global economy. Plant ailments, however, can reduce crop yields and result in financial losses. An automated artificial intelligence (AI)-based method for the automatic identification of plant diseases using resource-constrained Internet of Things (IoT) devices has been presented to solve this issue. However, the deployment of state-of-the-art convolution neural networks (CNNs) and Vision Transformers (ViT) on IoT devices is not feasible due to their large number of trainable parameters. To overcome this limitation, a meta-ensemble of lightweight MLP-Mixer and faster Long Short-Term Memory (LSTM) models has been proposed for plant disease detection on low-powered micro-controllers (MCUs) of IoT devices. The MLP Mixer model is based on a simple multi-layer perceptron network. The proposed meta-ensemble consists of two levels: predictions made by the trained models at the first level are used to train the machine learning classifier at the next level, resulting in further improvement of categorisation accuracy. The proposed meta-ensemble has been tested on three diverse datasets of varying sizes and plant species, including Maize, Cotton, and a dataset derived from the Plant Village(PV) dataset. On the Maize, Cotton, and derived PV datasets, respectively, experimental results demonstrate that the suggested technique obtained classification performance of 94.27%, 98.43%, and 97.45%. Moreover, prediction time of the proposed meta-ensemble is low, and it has considerably fewer trainable parameters than CNN and other transformer-based architectures. Therefore, the proposed meta-ensemble is an efficient and effective solution for plant disease detection with limited resources.
First Page
28096
Last Page
28108
DOI
10.1109/ACCESS.2024.3367443
Publication Date
1-1-2024
Recommended Citation
Maurya, Ritesh; Mahapatra, Satyajit; and Rajput, Lucky, "A Lightweight Meta-Ensemble Approach for Plant Disease Detection Suitable for IoT-Based Environments" (2024). Open Access archive. 7215.
https://impressions.manipal.edu/open-access-archive/7215
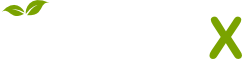
- Citations
- Citation Indexes: 13
- Usage
- Abstract Views: 4
- Captures
- Readers: 121
- Mentions
- News Mentions: 1