BADANet: Boundary Aware Dilated Attention Network for Face Parsing
Document Type
Article
Publication Title
IEEE Access
Abstract
Over the past few years, deep learning techniques have revolutionized the field of face parsing by utilizing massive datasets to generate high-level features and achieve outstanding performance. Usually, these techniques involve Convolutional Neural Networks (CNNs) to derive features from the input image and then a decoder network to forecast the semantic labels for every pixel. Even with complex deep convolutional neural networks (DCNN), incorrect parsing leads to a semantic gap between identical features, especially at boundary levels. Face parsing has encountered additional challenges due to the inclusion of pose, illumination and facial expressions. In order to address these issues, a Boundary Aware Dilated Attention Network (BADANet) is introduced which explores the use of multi-scale techniques to improve the accuracy and robustness of the per-pixel frames. BADANet's dilated attention module has been combined with a lightweight backbone to achieve exceptional results on LaPa, CelebAMask-HQ, and iBugMask. Extensive evaluations of the proposed method demonstrate its performance to be on par with various state-of-the-art techniques. The pipeline for the BADANet is available at https://github.com/abhigoku10/BADANet
First Page
106749
Last Page
106759
DOI
10.1109/ACCESS.2023.3319561
Publication Date
1-1-2023
Recommended Citation
Raghavendra, S.; Abhilash, S. K.; Nookala, Venu Madhav; and Srinidhi, N. N., "BADANet: Boundary Aware Dilated Attention Network for Face Parsing" (2023). Open Access archive. 8917.
https://impressions.manipal.edu/open-access-archive/8917
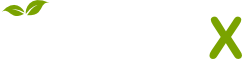
- Citations
- Citation Indexes: 1
- Usage
- Abstract Views: 2
- Captures
- Readers: 7
- Mentions
- News Mentions: 1