AI Trainer: Autoencoder Based Approach for Squat Analysis and Correction
Document Type
Article
Publication Title
IEEE Access
Abstract
Artificial intelligence and computer vision have widespread applications in workout analysis. It has been extensively used in sports and the athlete industry to identify errors and improve performance. Furthermore, these methods prevent injuries caused by a lack of instructors or costly infrastructure. One such exercise is the squat, which is a movement in which a standing person descends to a posture with their torso vertical and their knees firmly bent, then returns to their original upright position. Each person's squat is distinct, with varying limb lengths causing their form to change when observed. It has been observed that the mobility of various joints and muscular strength have a role in this. A squat improves the user by increasing overall leg strength, strengthening knee and hip joints, and lowering the risk of heart disease due to cardiovascular development. This paper presents a method for classifying squat types and recommending the right squat version. This study uses MediaPipe and a deep learning-based technique to decide if squatting is good or bad. A stacked Bidirectional Gated Recurrent Unit (Bi-GRU) model with an attention layer is proposed to consistently and fairly assess each user, categorizing squats into seven classes. This stacked Bi-GRU model with an attention unit is then compared to other cutting-edge models, both with and without the attention layer. The model outperforms other models by attaining an accuracy of 94% and is demonstrated to work the best and most consistently for our dataset. Furthermore, the individual executing the incorrect squat is corrected to the best of their ability, depending on their performance and body proportions, by providing the correct form.
First Page
107135
Last Page
107149
DOI
10.1109/ACCESS.2023.3316009
Publication Date
1-1-2023
Recommended Citation
Chariar, Mukundan; Rao, Shreyas; Irani, Aryan; and Suresh, Shilpa, "AI Trainer: Autoencoder Based Approach for Squat Analysis and Correction" (2023). Open Access archive. 8918.
https://impressions.manipal.edu/open-access-archive/8918
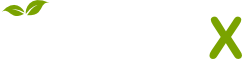
- Citations
- Citation Indexes: 12
- Usage
- Abstract Views: 2
- Captures
- Readers: 46
- Mentions
- News Mentions: 1